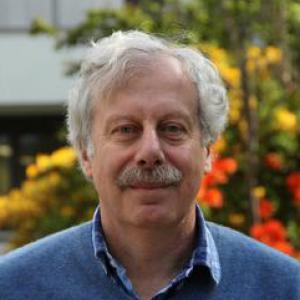
Vladimir Koltchinskii
Vladimir Koltchinskii is a professor in Mathematics at Georgia Tech. His current research is primarily in high-dimensional statistics and probability.
Title
Estimation of Functionals of High-Dimensional and Infinite-Dimensional Parameters of Statistical Models: Bias Reduction and Concentration
Abstract
This mini-course deals with a circle of problems related to estimation of real valued functionals of high-dimensional and infinite-dimensional parameters of statistical models. In such problems, it is of interest to estimate one-dimensional features of a high-dimensional parameter represented by nonlinear functionals of certain degree of smoothness defined on the parameter space. The functionals of interest could be often estimated with faster convergence rates than the whole parameter (sometimes, even with parametric rates).
We will discuss some mathematical methods providing a way to develop estimators of functionals of high-dimensional parameters with optimal error rates in classes of functionals of some H\"older smoothness and even to provide their efficient estimation with parametric rates when the smoothness is sufficiently large. The main focus will be on functionals of unknown covariance operators in high-dimensional and infinite-dimensional Gaussian models, where the functionals of interest often capture their spectral properties. In particular, we will discuss the role of higher order bias reduction methods and concentration inequalities in these problems.
The following topics will be discussed:
- Non-asymptotic bounds and concentration inequalities for sample covariance in high-dimensional
and dimension-free frameworks; - Some approaches to concentration inequalities for smooth functionals of statistical estimators;
- Higher order bias reduction methods in functional estimation (iterative bias reduction based on bootstrap chains; linear aggregation of plug-in estimators and jackknife estimators; methods based on Taylor expansion and estimation of polynomials with reduced bias);
- minimax lower bounds in functional estimation.
Lecture notes
The lectures' slides are available here.
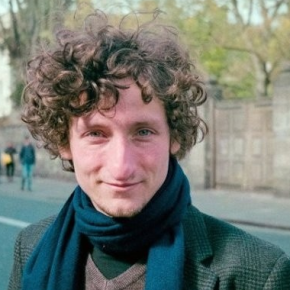
Bruno Loureiro
Bruno Loureiro is a CNRS research scientist at the Computer Science department on École Normale Supérieure (ENS) in Paris, working on the crossroads of machine learning and statistical mechanics. He holds a PhD degree in Physics from the University of Cambridge, and before moving to ENS he held postdoctoral positions at the École Polytechnique Fédérale de Lausanne (EPFL) and the Institut de Physique Théorique (IPhT) in Paris. He is interested in Bayesian inference, theoretical machine learning and high-dimensional statistics more broadly. His research aims at understanding how data structure, optimization algorithms and architecture design come together in successful learning.
Title
A Statistical Physics Perspective on the Theory of Machine Learning
Abstract
The past decade has witnessed a surge in the development and adoption of machine learning algorithms to solve day-a-day computational tasks. Yet, a solid theoretical understanding of even the most basic tools used in practice is still lacking, as traditional statistical learning methods are unfit to deal with the modern regime in which the number of model parameters are of the same order as the quantity of data – a problem known as the curse of dimensionality. Curiously, this is precisely the regime studied by Physicists since the mid 19th century in the context of interacting many-particle systems. This connection, which was first established in the seminal work of Elisabeth Gardner and Bernard Derrida in the 80s, is the basis of a long and fruitful marriage between these two fields.
The goal of this mini-course is to provide an in-depth overview of these connections and a good vision of the different tools available in the statistical physics toolbox, as well as their scope and limitations.
Note: No prior knowledge of statistical physics is expected.
Bibliography: The mini-course is based on the following lecture notes: https://brloureiro.github.io/assets/pdf/NotesPrinceton_BL.pdf
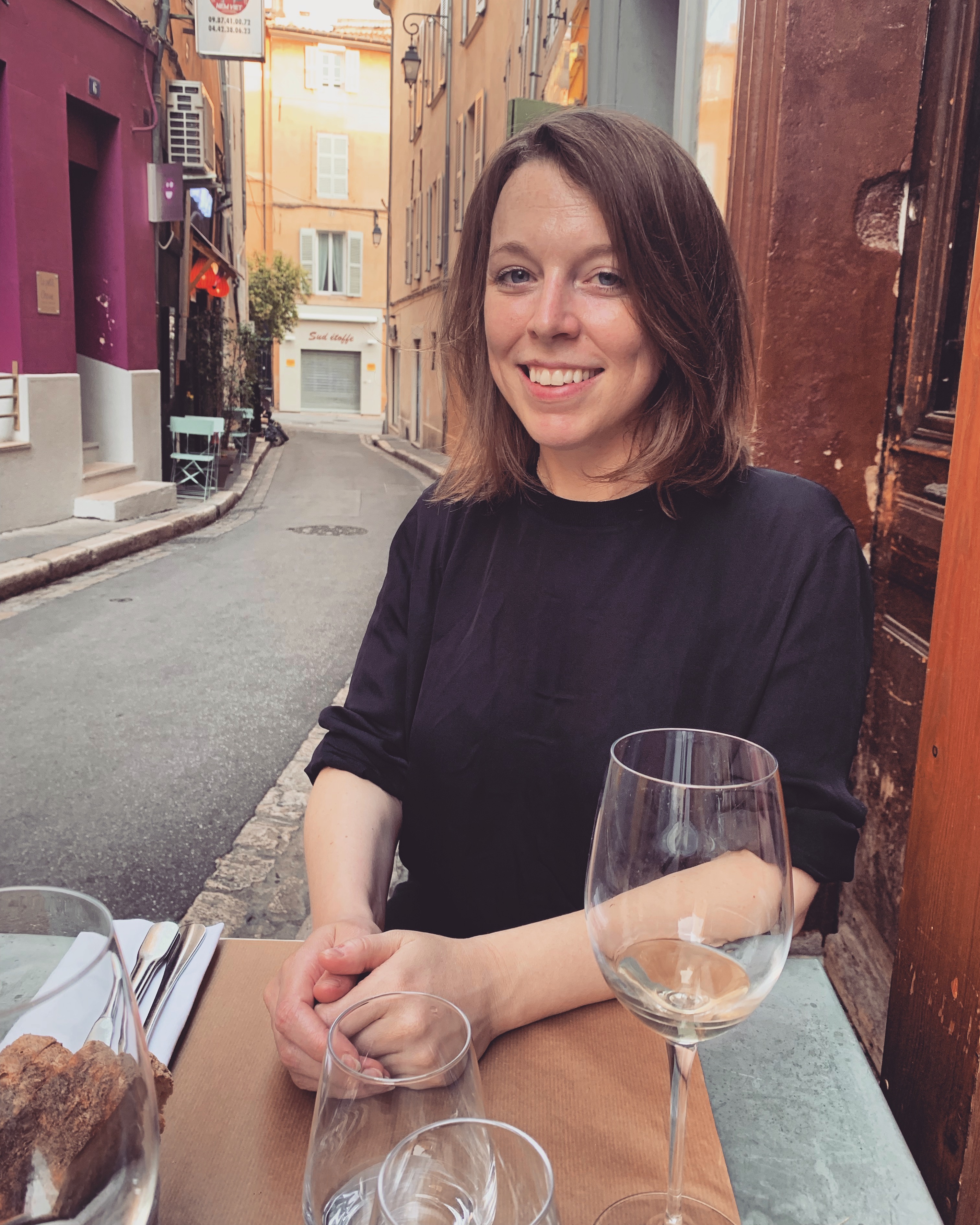
Cynthia Rush
Cynthia Rush is an Associate Professor of Statistics in the Department of Statistics at Columbia University. She received her Ph.D. in Statistics from Yale University in 2016, under the supervision of Andrew Barron. She obtained her B.S. in Mathematics at the University of North Carolina at Chapel Hill.
Her research uses tools and ideas from information theory, statistical physics, and applied probability as a framework for understanding modern, high-dimensional inference and estimation problems and complex machine learning tasks that are core challenges in statistics and data science.
To find out more about Cynthia's research and activities, you may visit her website here.
Title
High-Dimensional Statistics and Approximate Message Passing
Abstract
In this course, we will introduce the notion of high-dimensional statistics where one wishes to perform statistical prediction or inference in settings where the sample size of the data is smaller than or comparable to the number of parameters in the problem. In such settings, classical asymptotics and standard statistical methods can fail in unexpected ways. We will include a special focus on approximate message passing (AMP), which is a class of efficient, iterative algorithms that have been successfully employed in many statistical learning tasks like high-dimensional linear regression and low-rank matrix estimation. AMP algorithms have two features that make them particularly attractive: they can easily be tailored to take advantage of prior information on the structure of the signal, such as sparsity, and under suitable assumptions on a design matrix, AMP theory provides precise asymptotic guarantees for statistical procedures in the high-dimensional regime. In this course, we will cover the main ideas of AMP from a statistical perspective to illustrate the power and flexibility of the AMP framework and look at its application to matrix estimation.

Matus Telgarsky
Matus Telgarsky is an Assistant Professor at the Courant Institute of Mathematical Sciences at New York University, specializing in deep learning theory. He was fortunate to receive a PhD at UCSD under Sanjoy Dasgupta. Other highlights include: co-founding, in 2017, the Midwest ML Symposium (MMLS) with Po-Ling Loh (while on faculty at the University of Illinois, Urbana-Champaign); receiving a 2018 NSF CAREER award; and organizing two Simons Institute programs, one on deep learning theory (summer 2019), and one on generalization (fall 2024).
To find out more about Matus' research and activities, you may visit his website here.
Potential topic
Neural networks theory.